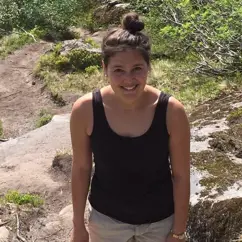
tjul@uni.gl | |
Telefonip normua | |
Immikkoortortaqarfik | Peqqissaanermik Pinngortitamillu Ilisimatusarfik |
Najugaq |
Ilimmarfik, Manutooq 1 Postboks 1061 3900 Nuuk |
The use of artificial intelligence and telemedicine solutions, to assess diabetic eye diseases in Greenland.
According to population health surveys conducted since 1999 10% of the Greenlandic population has type 2 diabetes. Several genetic traits, including the TBC1D4 gene, have been identified and been associated with insulin resistance. However, long-term complications from diabetes, such as diabetic retinopathy (DR), have not yet been investigated in Greenland. The aim of this PhD study is (1) to test if deep learning based automatic grading can replace manual ophthalmological grading of retina fundus photos using Optos ultra-wide-field cameras, (2) to estimate the incidence of diabetic eye disease in individuals with diabetes and pre-diabetes, and (3) to investigate whether monogenetic diabetes forms, primarily the TBC1D4 gene, are associated with higher incidence of DR, and investigate if diet and physical activity modifies the genetically increased risk.
The study population consists of participants identified with diabetes or pre-diabetes in previous or on-going population health surveys (approximately 700 persons), who are invited for complications screening including eye screening using the Optos camera in the current Greenland population health survey conducted from 2017-2019. All retina fundus photos are graded by trained ophthalmological nurses and an ophthalmologist and graded by an automatic grading algorithm developed using neural networks. Incidence of DR will be assessed using Cox- and Poisson regression models and methods for grading retina photos will be compared using Bland-Altmann plots and the variation will be assessed using a variance component model. The effect of the TBC1D4 variant and the potential modifying effect of lifestyle is modelled in a linear mixed effects model assuming a recessive interaction structure.